Clinical trials are a key area in which the use of AI in healthcare data has seen significant growth, yet this introduces unique legal challenges. AI development often relies on data collected from clinical trials to train algorithms, requiring careful consideration of consent, data origin and ethical standards. When data is acquired from third-party sources, transparency about collection methods, geographic origin and anonymisation standards becomes critical. While consent forms used in clinical trials can offer clearer terms for data use, ambiguity remains about how this data can be reused for AI purposes after the trial ends.
One of the main concerns involves data re-identification, in which anonymised data can potentially be traced back to individuals, especially when linked with other datasets. Data ownership is also a complex and often ambiguous area within the healthcare sector, particularly in the US. AI developers must clearly explain the value of data collection to hospital cybersecurity teams, ensuring they understand how the data will be used securely and ethically.
Data accuracy, fairness and robustness are key
The European Union’s AI Act presents a compelling model for regulating AI by adopting a risk-based approach. Under this framework, healthcare data falls into a “high-risk” category, necessitating stringent quality controls and ethical standards. This approach emphasises data accuracy, fairness and robustness, addressing many concerns surrounding the ethical implications of using AI in healthcare. The risk-based model is particularly well-suited to managing the complexities of health data, ensuring a high standard of data quality without impeding innovation.
Cybersecurity is another critical issue, as health data is particularly vulnerable to breaches, which can lead to identity theft, fraud and other risks. Ethical concerns also come into play, as improper use of health data can result in negative public reactions and erode trust in both healthcare providers and AI technologies.
Given these complexities, experts at the panel session: Unlocking Health Data: Navigating the Legal Landmines for Innovation during the MEDTECH 2024 Conference held in Toronto, Canada, offered several recommendations.
Clear ownership rights allow responsible data-sharing
Developing clear data ownership frameworks is essential. Establishing precise guidelines around data ownership, particularly for clinical trials and healthcare provider data, will promote transparency and reduce conflicts over data usage. Clear ownership rights can provide a foundation for responsible data-sharing practices.
How well do you really know your competitors?
Access the most comprehensive Company Profiles on the market, powered by GlobalData. Save hours of research. Gain competitive edge.
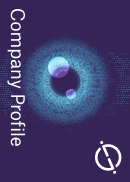
Thank you!
Your download email will arrive shortly
Not ready to buy yet? Download a free sample
We are confident about the unique quality of our Company Profiles. However, we want you to make the most beneficial decision for your business, so we offer a free sample that you can download by submitting the below form
By GlobalDataEnhanced communication with healthcare providers is equally crucial. AI developers should engage in open communication, explaining technical and regulatory terms in a way that is accessible to hospital staff and cybersecurity teams.
Adopting a risk-based regulatory approach, similar to the EU AI Act, would create a flexible yet high-standard regulatory environment. This approach would classify health data use as high-risk, enforcing stringent controls while still fostering technological advancements.
Public education on data security and ethics is also vital. Educating the public on data security practices and ethical AI use can help build trust and address common concerns about data privacy and AI. Public awareness initiatives can clarify why certain data is collected and how it is protected, further reinforcing confidence in these technologies.