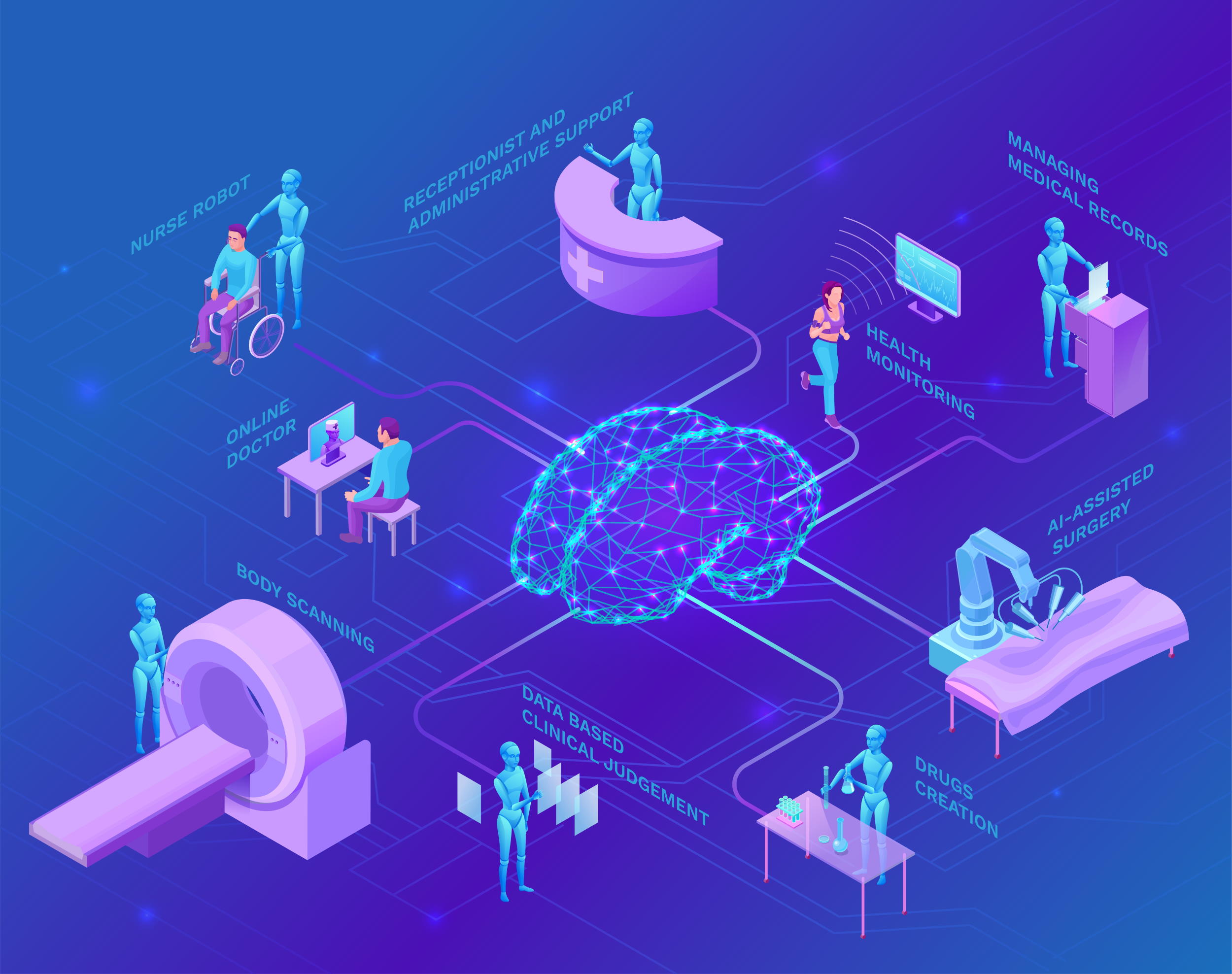
In common with virtually every other sector, the healthcare industry is being steadily transformed by artificial intelligence (AI) and machine learning. Just as AI is changing the way we design our cars, optimise our energy usage and handle our finances, so too is it bringing new opportunities – and risks – to the management of human health, from the potential of deep learning and neural networks in drug discovery to the growing sophistication of AI-enhanced diagnostics and surgical robotics.
Tying all this together is AI’s ability to bring together the vast oceans of data that permeate every aspect of healthcare to help inform decision-making among the sector’s key players, from pharmaceutical innovators to healthcare providers.
While AI and machine learning have become ubiquitous across all sectors – both as buzzwords and as increasingly practical solutions to persistent problems – their adaptation to healthcare inspires particular emotion. After all, few patients relish the idea that a machine might take responsibility for their diagnosis from a human doctor, despite the fact that AI-driven software now routinely outperforms humans in key diagnostic tasks. Others – most notably the esteemed cardiologist and researcher Dr Eric Topol – have countered that smart integration of AI could help make healthcare more human, not less.
As the conversation around AI in drug development and medical technology continues to evolve, the topic has of course been a persistent obsession across our websites. Here, we present some of the key trends and highlights from our coverage of AI in 2021.
The investment landscape in healthcare AI
Industry data is increasingly showing that the pharma and biotech sectors have been stepping up their investment in artificial intelligence and big data technologies in recent years. While a GlobalData (GD) survey suggested that AI and data analytics-related investments declined slightly in 2020 as the pandemic forced companies to focus on more urgent needs, interest is likely to resurge as the sector returns to a more business-as-usual footing in the coming years, as GD analyst Urte Jakimaviciute told Clinical Trials Arena last month.
How well do you really know your competitors?
Access the most comprehensive Company Profiles on the market, powered by GlobalData. Save hours of research. Gain competitive edge.
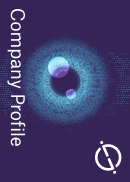
Thank you!
Your download email will arrive shortly
Not ready to buy yet? Download a free sample
We are confident about the unique quality of our Company Profiles. However, we want you to make the most beneficial decision for your business, so we offer a free sample that you can download by submitting the below form
By GlobalDataAnd the long-term trends for AI-related investment in the life sciences do look encouraging across a number of metrics. As our analysis of GD data has shown, AI-related financing deals jumped from 14 in Q1 2019 to 56 in the same quarter of 2021, while hiring for AI roles and the mentions of AI in company filings have also soared. The number of AI-based patents being generated by the pharma sector also suggests a healthy innovation eco-system, with AI patents rising from 20 in 2014 to 75 in 2020.
Based on the number of AI-related deals, job listings, patents and filings, GD has picked out top performers (or ‘MVPs’) in the AI space by sector. In pharma, Bayer, Novartis, Sanofi and AstraZeneca are among the AI leaders, while Philips, Medtronic, Thermo Fisher and Roche are some of the dominant AI players in the medical technology space.
AI continues to impress in drug discovery and design
But where exactly are these investments going? For biopharma players, AI is becoming an increasingly prevalent tool in drug discovery, design and target identification. UK-based AI drug discovery and design firm Exscientia hit a new milestone in 2021 with the Phase I trial of its second AI-designed molecule, and the world’s first for immuno-oncology. The promise of the company’s technology led to a $100m Series C funding round in March.
“Drug discovery is essentially a learning challenge and by learning more rapidly our AI systems are able to complete the discovery phase of each project with higher precision and much faster than traditional human-led approaches,” Exscientia CEO Andrew Hopkins told Clinical Trials Arena in April.
Exscientia is far from alone in the AI-driven drug discovery space, with the market bursting at the seams with innovative start-ups, including Montreal-based Valence Discovery, which is aiming to capture the clinical insights of what it calls ‘few-shot learning’. No surprise, then, that industrial giants such as Baidu CEO and founder Robin Li have argued that now is the time for AI and biocomputing in the quest to discover new drugs. The computing resources for such endeavours, meanwhile, are only growing – a perfect example is NVIDIA’s Cambridge-1, the UK’s most powerful supercomputer, which launched this year to help researchers, including those from AstraZeneca and GlaxoSmithKline, solve pressing medical challenges.
From sales to safety: embedding AI into operations
As the algorithms and their applications continue to evolve, AI and machine learning is being seeded ever more deeply into the operations of healthcare providers and innovators of all stripes. For example, the roadblocks that the Covid-19 pandemic has thrown up in the area of pharma sales – with reps forced out of in-person meetings and on to Zoom – are being tackled in part by AI-driven software that crunches huge reams of relevant data and comes back with actionable insights, reducing the manual groundwork for sales teams that have found themselves increasingly stretched to maintain productivity.
In drug safety, too, the pandemic has thrown down the gauntlet to regulators, public safety schemes and drugmakers to monitor the long-term impacts of new drugs and vaccines – often approved quickly under emergency provisions – at an unprecedented scale. AI has played an important role in this scale-up.
“The MHRA [UK Medicines and Healthcare products Regulatory Agency] updated their Yellow Card Scheme, and they attached a significant amount of artificial intelligence to that so patients could directly report to them,” IQVIA’s VP and global head of lifecycle safety Annette Williams told Pharmaceutical Technology in September. “The Yellow Card had been in place for a long time in the UK, but with Covid coming, they anticipated the need for speed.”
AI is driving the modern hospital (and home)
AI-enhanced medical technologies, meanwhile, now suffuse countless elements of the modern hospital, from smart robotic surgery to the way that patients can be triaged to prioritise those who need urgent hospital care over those who can afford to seek less acute treatment. Over time, machine learning, with its ability to draw from enormous data-sets, is likely to inform clinician decision-making in an ever-wider sphere. In May, Medical Device Network talked to Alife, a San Francisco-based start-up looking to leverage machine learning to optimise the delivery of in vitro fertilisation.
The home setting is undergoing AI-driven transformations, too, with platforms such as Cognetivity’s smartphone app OptiMind using pattern recognition algorithms to help users identify cognitive decline at the earliest opportunity. Some of the more radical AI interventions we covered in 2021 point to a not-too-distant future in which diagnostics are embedded into our most intimate appliances – as proved by the in-development ‘smart toilet’, which analyses human faeces as it’s flushed.
“The question we posed was: is there any health data in this solid waste that would normally go to the drain?” Duke University associate research professor Sonia Grego told Medical Device Network. “And sure enough, the answer is a resounding yes. There is plenty of health data that is not being captured because of people’s universal aversion to dealing with the specimen.”
AI is becoming a key enabler in clinical trials
The way that clinical trials manage recruitment, data analysis and study design is increasingly being influenced by AI and the growing group of providers that are offering algorithms to help optimise trials. Optimised matching of patients with trials is increasingly in-demand as trials become more diverse and decentralised, while AI is also helping to drive simulation and modelling capabilities that could transform the way that clinical studies are designed.
And just as smart diagnostics have made their presence felt in clinical practice, they are also having an impact on the analysis of clinical trial data. In October, Clinical Trials Arena reported on Sagimet Biosciences’ use of AI-powered tools for liver pathology assessment in its Phase IIb trial in non-alcoholic steatohepatitis (NASH), with artificial intelligence helping to sidestep bias issues among human pathologists when they examine images of biopsy samples.
AI issues could spell trouble in paradise
It’s not all plain sailing in AI world, however. The increasing incorporation of artificial intelligence into healthcare and pharmaceutical processes brings with it complex challenges of its own, some of which we delved into this year. For example, while Sagimet’s aforementioned use of AI-powered image analysis is intended to counter human bias, if machine learning algorithms are built without due care, they are perfectly capable of carrying over biases themselves, as one University of Chicago study showed in June. Technical flaws can also cause AI to undervalue certain factors, as may be the case in disease prediction modelling.
Nevertheless, responsible building and management of AI can help to mitigate these biases, Cognoa chief medical officer Dr Sharief Taraman argued in August. “We need to be thoughtful about how we apply AI and make sure that we have diverse training datasets,” he said. “When we apply the AI we need to continue to monitor it and use it not as a replacement for physicians but instead as something that adjuncts their abilities.”
Perhaps most challenging of all is the prospect of regulating AI-based technologies, which still represent uncharted regulatory territory in many cases. Regulators will need to be agile in their approach or risk stumbling in their tightrope walk between ensuring quality and encouraging innovation.
“There are aspects of AI that are very radically different, particularly the ability of the software to learn and improve with real-world data that it encounters,” medical device lawyer Jeffrey K. Shapiro told Medical Device Network earlier this year. “I don’t think the FDA has any idea how to regulate that, because it’s totally outside the usual paradigm of developing a medical device, validating it, marketing it after FDA review and then the performance characteristics don’t change. Here, by definition, they are supposed to change and the FDA hasn’t figured out how they’re going to handle it yet.”
This article is part of a special series by GlobalData Media on artificial intelligence. Other articles in this series include:
Japan is leading the way into the elderly-population future. Its solution? AI-powered “Society 5.0”
Big Tech leads the AI race – but watch out for these six challenger companies
Financial sectors look to AI in site selection