Recently, artificial intelligence (AI) has been paving the way and helping sponsors with patient identification and participant retention. Digital twins, a new technological frontier in the AI space, is challenging the conservative nature of clinical trials.
In simple terms, a digital twin is a copy of a biological entity, such as a cell, an organ, or a person. This digital replica allows researchers to observe the patient in real time while simulating and predicting a different clinical outcome for the patient in a clinical trial.
While the term originates from the jet engineering industry, where computer models are used to simulate how a jet engine would operate in different scenarios, the human body is more complex and does not have a similar blueprint. Due to regulatory uncertainty, digital twins are yet to prove their value, but their potential to change the course of clinical trials is certain.
How do digital twins work?
The aim of a clinical trial is to measure the difference between what happens when a patient receives the experimental treatment and what would happen if they did not. However, both outcomes cannot be observed in one patient. Digital twins allow researchers to ask what-if questions about real people in clinical trials, explains Charles Fisher, PhD, founder and CEO at Unlearn. When using this technology, there can be both an observed outcome and an estimated outcome.
Data is the key element of creating a digital twin, and this largely defines how successful it will be when deployed in clinical trials, adds Stefan Harrer, PhD, chief innovation officer at Digital Health CRC. Ideally, one would need everything from the genome to the exposome, meaning from the inner building blocks of the cell all the way to the environmental factors.
To get a hold of such data is challenging, but with electronic health records, data linkage, and DNA sequencing becoming more of a commodity, there is a sufficient body of data that allows the building of digital twins, Harrer explains. With such strict data requirements, certain therapy areas like oncology and neurological diseases could lend themselves better to the creation of digital twins.
How well do you really know your competitors?
Access the most comprehensive Company Profiles on the market, powered by GlobalData. Save hours of research. Gain competitive edge.
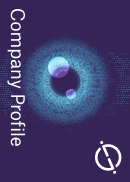
Thank you!
Your download email will arrive shortly
Not ready to buy yet? Download a free sample
We are confident about the unique quality of our Company Profiles. However, we want you to make the most beneficial decision for your business, so we offer a free sample that you can download by submitting the below form
By GlobalDataDigital twins are not replacing people
A common misconception with digital twins is the assumption that they will represent the person close enough, says João Bocas, CEO of digital health company Digital Salutem. “There will be always a level of a discrepancy because it is impossible to be 100% right in copying a person.”
Computer models can be wrong, so a randomised control trial (RCT) approach is still needed. For example, there might be patients who are receiving the actual control drug and digital twins who are predicting how a patient would respond if they got the control. The actual patient data allows researchers to recalibrate the analysis and ensure the statistical properties, Fisher explains.
Another misconception is that not all participants require a digital twin. Whether they are in the treatment arm or control group, every single patient in all of the cohorts needs to have a digital twin, otherwise the trial is no longer blinded.
Lack of regulatory oversight
When it comes to the regulatory framework, there is uncertainty about how digital twins are regulated, and it all depends on the use case. Certain statistical methods are acceptable to the FDA and EMA, Fisher explains, adding that Unlearn received EMA qualification back in September. However, the FDA does not have the same qualification process or sufficient resources to do the reviews. “Within the United States, we are effectively unregulated, but there is broad high-level statistical guidance from the FDA and our methods apply to them,” he adds.
The FDA has a variety of programs that, in theory, look at the use of models in drug development, such as the Complex Innovative Trial Design Meeting Program and Model-Informed Drug Development Paired Meeting Program. Unlearn has been in contact with the agency, but these programs are under-resourced. Both the EMA and FDA charge user fees to review drugs, but only the EMA has a pathway that reviews software tools or models and charges user fees to financially support these reviews.
Even though the EMA is typically more conservative than the FDA, the successful utilisation of digital twins depends on money and resources. “The EMA has it and the FDA does not. I hope in the future Congress will fix [this issue] and provide the FDA with an avenue to get more resources for this type of regulation, which is going to be very important in the future,” says Fisher.
Regulatory guidance and policies are needed to ensure the right use of digital twins, especially due to the use of patient data. Patient consent is also a challenge as the data for creating digital twins comes from previous trials, Harrer adds. “If patients have given their consent to their data being used as part of a previous trial, does that automatically mean their data can be used to create digital twins?” he questions. It is also crucial to ensure that the data selection does not amplify potential biases from previous data sets.
However, regulators do acknowledge the potential of digital twins because this technology provides the same statistical characteristics that they are looking for, Fisher says. “This is a rare win-win technology that benefits everybody involved,” he adds.
Benefits to participants
A digital twin can offer several benefits to trial participants. First, it allows researchers to personalise treatments and gain insight on how to treat the condition better. “If we can replicate a specific condition, we can target the condition in a more efficient and agile way,” says Bocas.
On ClinicalTrials.gov, two ongoing trials are validating digital twins, specifically one to enable precision treatment for patients with type 2 diabetes (NCT05181449) and one on strength training for musculoskeletal injuries and diseases (NCT04849923). Two completed trials have evaluated digital twins in insulin delivery and meal prediction algorithms (NCT04203823) and giving personalised dietary advice by predicting postprandial responses (NCT05313594).
Digital twins also help with patient recruitment. Participant eligibility, suitability, and motivation create a huge bottleneck for trial enrollment and carry some of the highest cost burden, which only gets amplified in the rare disease space, says Harrer.
Although clinical trials are not there to deliver treatments, the prospect of not getting the experimental drug has proven to be an inhibitor for patients enrolling in these trials. Digital twin technology can be used to create a part of the control group so that more patients will receive the treatment.
There is a strong possibility that digital twins may lead to shorter clinical trial timelines, which could be reduced by six months or one year, according to Fisher. This would open the door for faster development of medication and new treatments for people with rare diseases, Harrer notes.
Time to act now
While it is certain that AI and digital twins will not replace real patients or doctors, people who are not in favour of the technology might miss out in the future, Bocas says. “It is more about the evolution of medicine, and those who are using this technology will certainly have an advantage,” he adds.
Although digital twins are a new technology with many unanswered questions, sponsors must take a measured risk to find out if it works. However, the pharmaceutical industry is conservative and risk-averse, Bocas explains, adding that fears of failure or loss of money work against innovation.
In the clinical trials space, there tends to be a philosophy of not sharing, and even failed clinical trial data is one of the most powerful but least used resources in pharmacological research, says Harrer. “For this technology to really flourish and be impactful, it needs to be documented, shared, and communicated to the outside world,” he adds.
This is a highly interdisciplinary area. The responsible and successful development of digital twins requires deep data science, as well as digital and clinical trial experience. “This is not something that either one side can do by their own,” says Harrer. “All of these trials require a deep interlock between the world of data science and the clinical world.”