One pain point for sponsors is trial termination due to lack of recruitment, partially a result of recruiting the wrong principal investigators (PIs) and sites without the patient population required for the study. New York-based H1 reports that more than 80% of trials fail to enrol participants on time, leading to $75m per year in wasted costs. CEO Ariel Katz says his company aims to put an end to this by helping to connect the ‘world to the right doctors’.
H1 claims that Trial Landscape is a ‘first of its kind’ repository that incorporates global and local trial registries while also including diversity and inclusion insights, providing sponsors with more representative trial recruitment. The software connects sponsors with PIs and sites with a good patient population for the trial while also considering how diverse that patient population is.
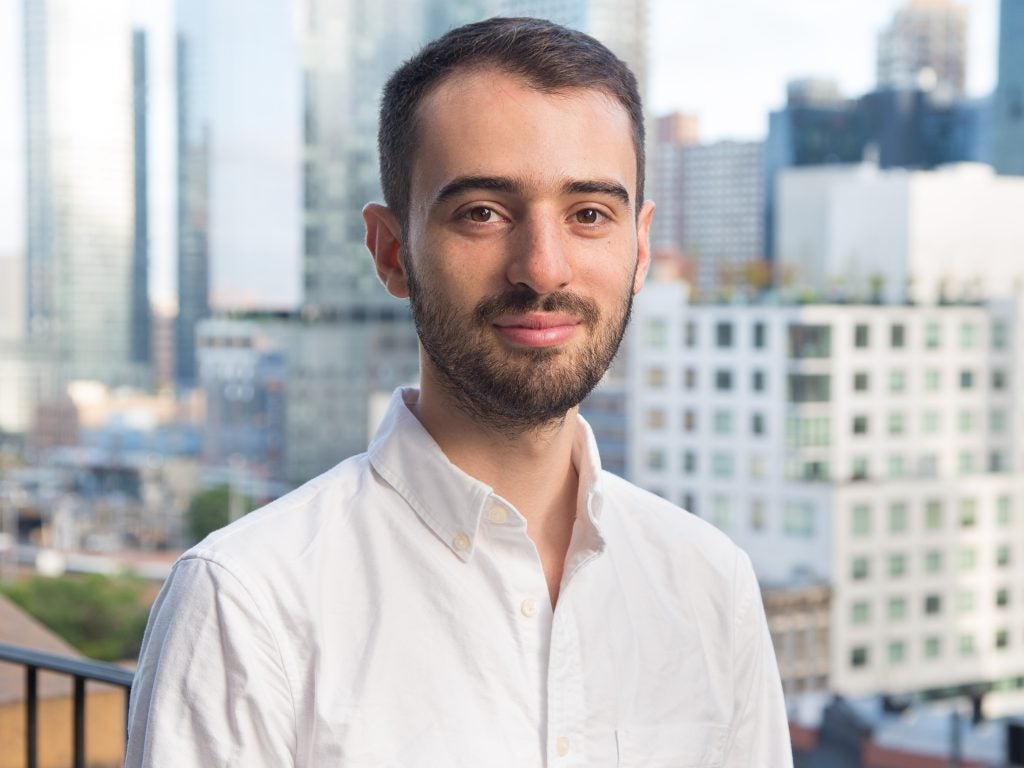
Abigail Beaney: How is your software benefiting sites, sponsors, CROs and patients?
Ariel Katz: H1's mission is to connect the world to the right doctors and one of the ways organisations connect patients to the right doctors is in clinical trials. Organisations need to find the right PI to work on a clinical trial to recruit patients and find the right site where those patient populations exist. Currently, it's a pretty broken process. The number of sites and PIs that are selected and don't recruit patients is staggeringly high from an outsider’s perspective. The way that process historically works is that smaller organisations rely on the CRO. Larger organisations also depend on the CRO, but they also have their own validation checks and recommend sites. The process is pretty manual - they're aggregating data sources, they're doing analysis and trying to pinpoint where patient populations are. It's a very difficult task and it's very manual but now there's more technology and data to solve it. With generative AI coming into the fold, technology is able to generate an answer by leveraging data sources, removing the human layer of manual curation. We are trying to push that technology and innovation towards industry because we think it's needed and we think it can save a lot of time, lead to better decisions, faster clinical trials and speed up bringing therapies to market.
AB: How does your software differ from other AI software that match patients up with trials?
AK: If you were trying to solve the problem of finding a site and a PI to work on a clinical trial, what would you do? You would say, who has worked at one in the past? You want to aggregate all that information about who has experience. You would then want to ask, who are the ones that have patients that fit the criteria that I need? So that's important too. Then you want to ask, who were the ones that performed well on it in the past? Then you might get into more specific questions like, do they work with my competitors? Are they really going to work with me if I'm a smaller company? Can I afford them? Those are some of the questions you'll ask. Another question that became really important is, do they see diverse patients? Do they have diverse patient populations? And that's really where H1 started, and with Trial Landscape you can answer that question now. You might already have data to know if they have patients, how they have performed in the past, and where they work. With Trial Landscape, you can ask questions like do they have diverse patients? That is where we started in the clinical trial space. We then expanded to let users ask how well PI's did in previous clinical trials. For example, they may have worked on a clinical trial with a big Pharma company but did they recruit any patients? Were there protocol violations? How well did they recruit and how quickly? Now you know who sees diverse patients, who worked on those clinical trials and how they performed. The next question you want to ask is, can you just tell me who to pick? Can I just input all my information and let the computer find the answer for me? That is where AI comes into place. We believe and we've seen that computers are better at answering that question than humans because they can process more data than we can.
AB: Is the addition of diversity questions one of the selling points of your software?
AK: Without AI you need to make sure that you are manually fulfilling the diversity needs. AI is going to supercharge that and make it much easier. If you think about diversity, it's a complex thing. What is the definition of diversity in a clinical trial? What do you think about low- and middle-income communities versus race and ethnicity? What do you think about diversity in the UK? The NHS differentiates between Irish and British. In New York we don't differentiate between Irish and British. So what do you think about diversity in different regions? There's no human being that knows the definition of ethnicity in every country around the world because it's a very complex thing to do. We think the diversity information we have layered with AI gets an answer much quicker and is much more precise, globally, than a human being. A human may not physically be able to process that data or it may take forever and it will be imperfect so that's what we're doing in the industry.
AB: What limitations and difficulties have you faced in developing this software? And what ways have you combated these?
AK: If you wanted to aggregate information on the race and ethnicity of patients, how do you do that? It is such a big challenge. Getting access to information compliantly was a huge challenge and a hurdle we had to overcome. One example is gaining that information in the EU because of GDPR. But when you get that information, how do you then think about ethnicity in these countries as it is very different? In the US, diversity is very much around African American, Caucasian and Asian races. In the UK, it's very diverse; you have Pakistani, Irish, British, you have all these different ethnicities. In Brazil, it's very different too. Another challenge was, how do you think about the definitions of race and ethnicity and help educate pharma companies who have not historically thought about this that much when they're developing clinical trials? So, we've had to overcome that sensitive topic as well.
AB: One issue for clinical trials is the difficulty of meeting endpoints due to lack of patient recruitment. How has your software improved this?
AK: So, if a clinical trial picks 100 sites, 50% of them don't recruit a single patient. Trial Landscape can reduce that so that about half of those who are selected are able to recruit patients. That leads to a few different benefits. It costs a lot of money to set up a site so you don't want to spend that picking a site that won't recruit patients. The second thing that it does is if you pick the right site early, you don't need to rush to spin up a new site so you save time and drugs come to market quicker. Finding the right sites and PIs has a huge benefit for ROI with trials not taking as long and bringing drugs to market quicker.
AB: What are your hopes for the future of H1?
AK: I want to achieve a mission - I want to connect the world to the right doctors and I want to do that across the whole landscape. We want to help in every way from finding the right doctor to run a clinical trial to helping patients to find the right therapy and the right doctor. In five years, what you would ultimately want is a machine where you just input 'here's a clinical trial, here's the number of patients I want to enrol, here's how much money I have to spend, find me all the PIs and sites that fit these criteria that have these patients' and you just want an answer. We want to be able to bring that to the industry and we want that answer to be way more precise than what happens now. So trials can be quicker, and therapies can come to market quicker. That's what we want to do.