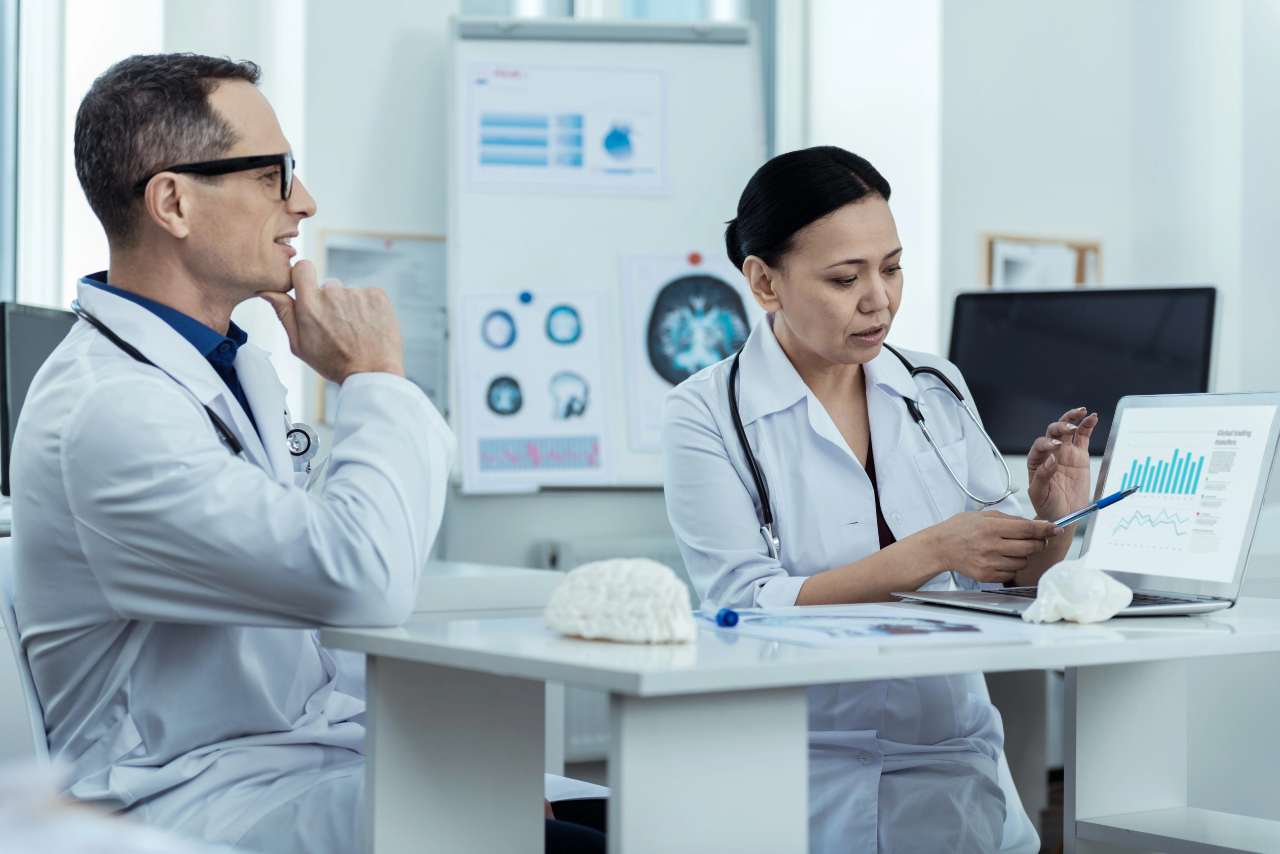
Traditionally, oncology trials have been designed to focus on the treatment of cancer in a specific location of the body. Advances in precision medicine have proven that patients with the same genetic mutation, regardless of cancer location or tumor type, have more in common than patients with the same tumor type and different genetic mutations.
Clinical trial design has shifted to accommodate these advances in oncology research. Basket trials are one of many clinical trial designs that seek to answer questions based on these understandings.
One of the potential benefits of basket trials is that they require less patients and shorter trial duration to identify an effective agent. However, statistical challenges still arise when pooling data across tumor types. As a potential solution, many sponsors are exploring Bayesian Futility Analysis as a way to better evaluate the Probability of Success (PoS) rates across treatment arms.
Implementing Bayesian Futility Analysis has the potential to decrease the likelihood of enrolling patients into treatments that are ineffective. Each time a new patient enrolled, the sponsor would need to determine how many evaluable patients were already enrolled in the study and how many of those patients were responding to treatment. This requires an immense amount of time and effort by statisticians to make the Bayesian algorithmic calculations manually every time new patients enroll in a study.
This is where IRT comes in. We built our IRT with focus on automating the complexities in oncology trial design to reduce manual calculations and provide built-in agility to dynamically manage the unpredictable mid-study adjustments often experienced.
So naturally, we asked ourselves “How can we successfully implement the Bayesian algorithm, and automate the calculations in our IRT system – to minimize risk, time, and costs for our clients?”
In order to do this, we worked closely with a sponsor to create an automated integration that ultimately enabled real-time data analysis and adjustment of cohorts, increasing the likelihood of enrolling patients into effective cohorts within their Basket Trials.
Here’s what it looked like:
To go into some more detail on this 2-way integration:
Integrating the Statistical Algorithm into IRT
This required both the IRT system and the statistical algorithm to openly send real-time data back and forth in order to determine which of the study’s cohorts should remain open or closed for enrollment, as well as determine which cohort a patient should be enrolled.
Automatic Patient Enrollment Using Real-Time Data
In the IRT system, patient enrollment into a cohort was using real-time data. Every time a patient was ready to enroll into the study, the IRT system would send the most up-to-date data automatically to the statistical algorithm to perform the calculations, and that patient would be enrolled into an open cohort. After a patient was enrolled into a cohort, the site user then entered 2 data points that would allow the IRT system to automatically determine the evaluability status of every enrolled patient and whether the patient was responding to treatment:
- If the patient had a scan or disease assessment
- If the patient had a response and was effectively improving
Takeaway: Building in Flexibility for Unknowns
Historically, oncology studies lend themselves to a variety of unpredictable mid-study changes that could result in costly delays. With this in mind, Suvoda’s IRT system was built so that the study team could easily update data points without the need for mid-study system change orders. For this innovative Bayesian integration, it was also important for the study team to have the immediate ability to manually override both the integration and patient evaluability/responder status, as well as the ability to quickly update incorrect site data – no change orders needed!
What could this mean for your study team?
- Faster study execution
- Decreased study team workload
- Overall cost savings
Ultimately, Suvoda exists to help sponsors bring life-saving treatments to patients. By reducing some of the complexities of basket trials through innovative integrations like this one, sponsors and CROs can rely on faster study execution so that their treatments can get to market and save lives as quickly as possible.